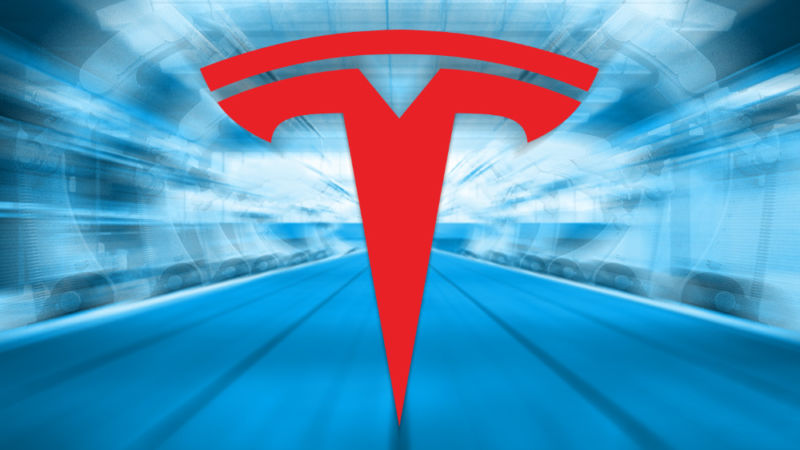
There's an old joke in the software engineering world, sometimes attributed to Tom Cargill of Bell Labs: "the first 90 percent of the code accounts for the first 90 percent of the development time. The remaining 10 percent of the code accounts for the other 90 percent of the development time."
On Monday, Tesla held a major event to show off the company's impressive progress toward full self-driving technology. The company demonstrated a new neural network computer that seems to be competitive with industry leader Nvidia. And Tesla explained how it leverages its vast fleet of customer-owned vehicles to collect data that helps the company train its neural networks.
Elon Musk's big message was that Tesla was close to reaching the holy grail of fully self-driving cars. Musk predicts that by the end of the year, Tesla's cars will be able to navigate both surface streets and freeways, allowing them to drive between any two points without human input.
At this point, the cars will be "feature complete," in Musk's terminology, but will still need a human driver to monitor the vehicle and intervene in the case of a malfunction. But Musk predicts it will only take about six more months for the software to become reliable enough to no longer require human supervision. By the end of 2020, Musk expects Tesla to have thousands of Tesla vehicles providing driverless rides to people in an Uber-style taxi service.In other words, Musk seems to believe that once Tesla's cars become "feature complete" later this year, they will be 90 percent of the way to full autonomy. The big question is whether that's actually true—or whether it's only true in the Cargill sense.
Two stages of self-driving car development
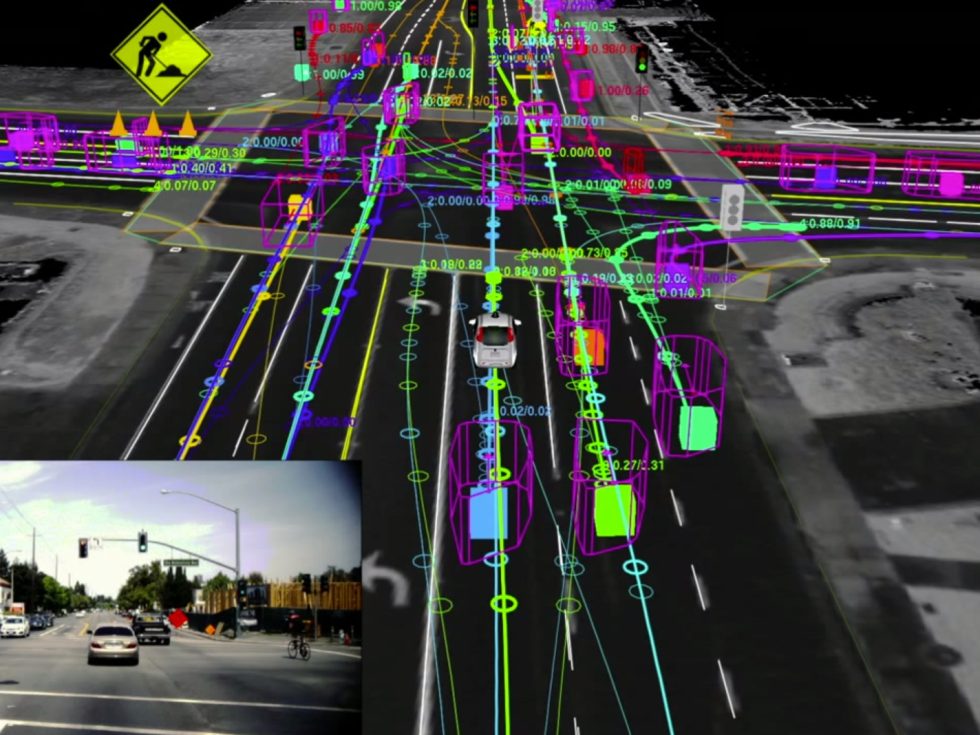
You can think of self-driving car development as occurring in two stages. Stage one is focused on developing a static understanding of the world. Where is the road? Where are other cars? Are there any pedestrians or bicycles nearby? What are the traffic laws in this particular area?
Once software has mastered this part of the self-driving task, it should be able to drive flawlessly between any two points on empty roads—and it should mostly be able to avoid running into things even on crowded roads. This is the level of autonomy Musk has dubbed "feature complete." Waymo achieved this level of autonomy around 2015, while Tesla is aiming to reach it later this year.
But building a car suitable for use as a driverless taxi requires a second stage of development—one focused on mastering the complex interactions with other drivers, pedestrians, and other road users. Without such mastery, a self-driving car will frequently get frozen with indecision. It will have trouble merging onto crowded freeways, navigating roundabouts, and making unprotected left turns. It might find it impossible to move forward in areas with a lot of pedestrians crossing the road, for fear one might jump in front of the car. It will have no idea what to do near construction sites or in busy parking lots.
A car like this might get you to your destination eventually, but it might be such a slow and erratic ride that no one wants to use it. And its clumsy driving style might drive other road users crazy and turn the public against self-driving technology.
In this second stage, a company also needs to handle a "long tail" of increasingly unusual situations: a car driving the wrong way on a one-way road; a truck losing traction on an icy road and slipping backward toward your vehicle; a forest fire, flood, or tornado that makes a road impassable. Some events may be rare enough that a company might test its software for years and still never see them.
Waymo has spent the last three years in the second stage of self-driving development. By contrast, Elon Musk seems to view it as trivial. He seems to believe that once Tesla's cars can recognize lane markings and other objects on the road that it will be just about ready for fully driverless operation.
Tesla’s new self-driving chip
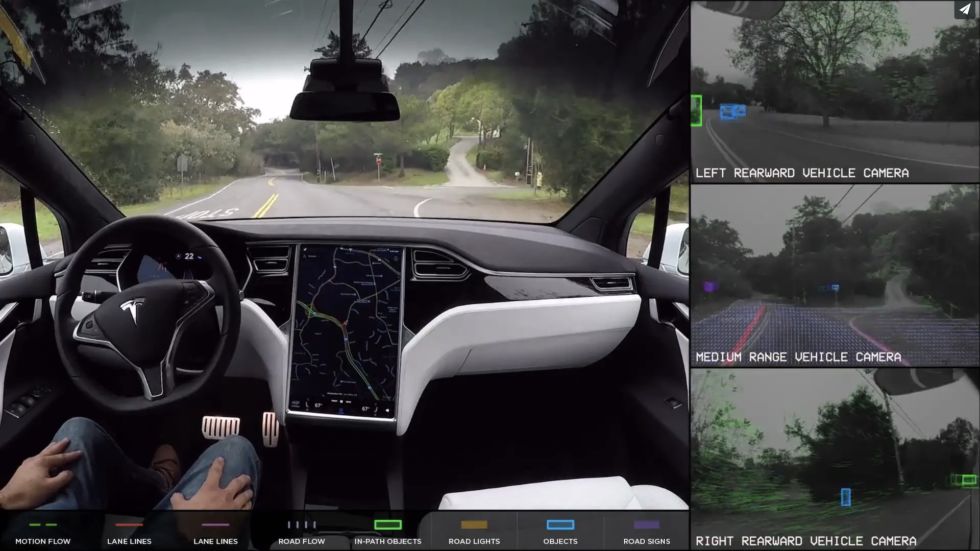
Over the last decade there has been a deep-learning revolution as researchers discovered that the performance of neural networks keeps improving with a combination of deeper networks, more data, and a lot more computing power. Early deep learning experiments were conducted using the parallel processing power of consumer-grade GPUs. More recently, companies like Google and Nvidia have begun designing custom chips specifically for deep learning workloads.
Since 2016, Autopilot has been powered by Nvidia's Drive PX hardware. But last year we learned that Tesla was dumping Nvidia in favor of a custom-designed chip. Monday's event served as a coming-out party for that chip—officially known as the Full Self-Driving Computer.
Musk invited Pete Bannon, a chip designer Tesla hired away from Apple in 2016, to explain his work. Bannon said that the new system is designed to be a drop-in replacement for the previous Nvidia-based system.
"These are two independent computers that boot up and run their own operating systems," Bannon said. Each computer will have an independent source of power. If one of the computers crashes, the car will be able to continue driving.
Each self-driving chip has 6 billion transistors, Bannon said, and the system is designed to perform a handful of operations used by neural networks in a massively parallel way. Each chip has two compute engines capable of performing 9,216 multiply-add operations—the heart of neural network computations—every clock cycle. Each Full Self-Driving system will have two of these chips, resulting in a total computing capacity of 144 trillion operations per second.
Tesla says that's a 21-fold improvement over the Nvidia chips the company was using before. Of course, Nvidia has produced newer chips since 2016, but Tesla says that its chips are more powerful than even Nvidia's current Drive Xavier chip—144 TOPS compared to 21 TOPS.
But Nvidia argues that's not a fair comparison. The company says its Xavier chip delivers 30 TOPS, not 21. More importantly, Nvidia says it typically packages the Xavier on a chip with a powerful GPU chip, yielding 160 TOPS of computing power. And like Tesla, Nvidia packages these systems in pairs for redundancy, producing an overall system with 320 TOPS of computing power.
Of course, what really matters isn't the number of theoretical operations a system can perform, but how well the system performs on actual workloads. Tesla claims that its chips are specifically designed for high performance and low power consumption for self-driving applications, which could yield better performance than Nvidia's more general-purpose chips. Regardless, both companies are working on next-generation designs, so any advantage either company achieves is likely to be fleeting.
https://arstechnica.com/cars/2019/04/teslas-autonomy-event-impressive-progress-with-an-unrealistic-timeline/
2019-04-25 15:14:00Z
52780273338642
Tidak ada komentar:
Posting Komentar